Open Source Tools for Securing Generative AI Systems
Join our weekly newsletters for the latest updates and exclusive content on industry-leading AI, InfoSec, Technology, Psychology, and Literature coverage. Learn More
The Rise of Open Source Security Tools for Generative AI
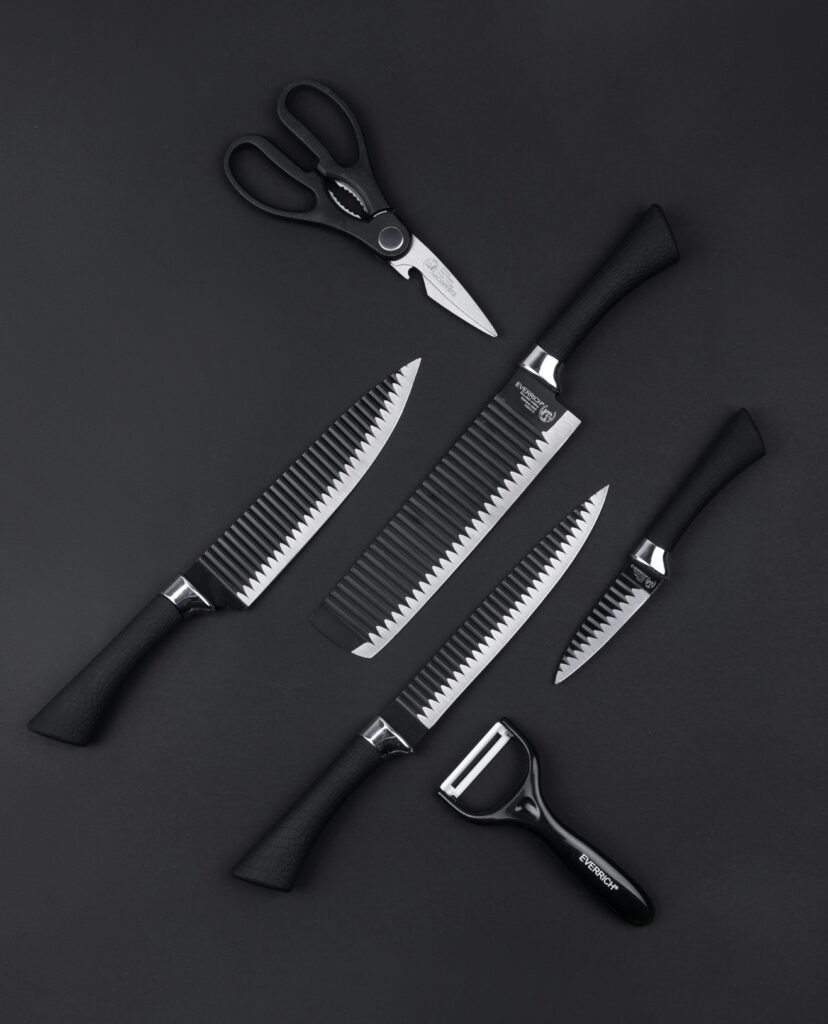
The increasing complexity of generative AI systems, particularly large language models (LLMs), has prompted a corresponding rise in the development of open source security tools tailored for their protection. As these systems become more integrated into various industries, the need for effective security measures has become paramount. Open source tools provide unique advantages, as they are often created through collaborative efforts from diverse stakeholders, including academic researchers and cybersecurity consultancies. This collective expertise enhances the robustness and adaptability of security solutions available for generative AI.
Innovative open source security tools, such as ‘Broken Hill’ and various prompt injection testing frameworks, have emerged as critical resources. These tools are specifically designed to identify and mitigate vulnerabilities inherent in generative AI systems. ‘Broken Hill,’ for instance, focuses on the analysis of model behavior, providing insights that can help organizations fortify their AI applications against potential threats. By employing such tools, companies can proactively assess the security posture of their generative AI implementations and address weaknesses before they can be exploited.
The role of AI security firms in this landscape is equally noteworthy. These firms not only contribute to the development of tools but also offer guidance on best practices for securing generative AI applications. Their expertise helps organizations understand the nuances of securing LLMs and other generative AI technologies that have become vital assets for many businesses. As the AI landscape continues to evolve, the integration of open source security tools and collaborative development will be essential to foster a secure environment for generative AI applications, ensuring they can operate safely and effectively.
Understanding Prompt Injection Attacks and Their Mitigation
Prompt injection attacks represent a significant threat to generative AI systems, exploiting the inputs provided to these models to manipulate their outputs. Such attacks can occur when adversaries craft inputs designed to confuse or deceive the AI, leading to undesired or potentially harmful responses. As businesses increasingly rely on generative AI, including chatbots and content generators, understanding these vulnerabilities is paramount for effective security measures.
Recent breakthroughs in attack techniques have further complicated the landscape of AI security. One notable approach is the greedy coordinate gradient (GCG) method, which effectively identifies the most impactful dimensions of the input that can be manipulated for a successful attack. By iteratively adjusting these dimensions, an attacker can devise inputs that yield specific, exploitative outcomes. Another innovative method is the tree of attacks with pruning (TAP), which systematically explores various attack vectors to detect the most promising paths for exploitation. This capability allows attackers to maximize their success rates while minimizing computational resources.
Additionally, a technique known as deceptive delight seeks to manipulate generative AI systems by leveraging misleading or contextually irrelevant inputs that produce unexpected yet charming outputs. This not only detracts from the reliability of AI-generated content but also poses reputational risks for organizations utilizing such systems. In light of these evolving attack strategies, businesses must prioritize the implementation of robust testing tools designed to uncover weaknesses in AI applications. Regular security audits and rigorous adversarial testing can help identify vulnerabilities and enhance the overall resilience of generative AI systems.
As the landscape of prompt injection attacks evolves, businesses must remain vigilant and proactive in their efforts to secure AI applications. By fostering a comprehensive understanding of these sophisticated threats and employing advanced testing methodologies, organizations can better safeguard their generative AI systems against exploitation.
Evaluating AI Guardrails with New Testing Frameworks
As organizations increasingly rely on generative AI systems to streamline their operations, the security of valuable business data is of paramount importance. The deployment of AI guardrails is essential to mitigate risks associated with these innovative yet vulnerable systems. With such concerns, the new testing frameworks developed to evaluate AI guardrails are becoming crucial tools in ensuring robust security measures. Among them, Microsoft’s PYRIT stands out as a highly effective solution aimed at simulating adversarial attacks, thereby rigorously testing the resilience of generative AI systems against potential exploits.
PYRIT is specifically designed to identify vulnerabilities that could be exploited through prompt injection and other attack vectors. By simulating various threat scenarios, it allows organizations to understand the effectiveness of their AI guardrails. However, it is imperative to compare PYRIT with other testing frameworks like PromptGuard and LlamaGuard as these solutions also offer vital insights into the strengths and weaknesses of AI security deployments. PromptGuard, for instance, focuses on facilitating a structured approach to testing prompt integrity, while LlamaGuard emphasizes comprehensive security assessments through a broader range of attack simulations.
When companies adopt these testing frameworks, they empower themselves to evaluate the efficiency of their defenses against jailbreak and similar risks actively. Regularly assessing the performance of AI guardrails allows businesses to refine their strategies, ensuring that generative AI systems remain secure, reliable, and efficient. The role of AI security firms in this landscape cannot be understated; they provide expert guidance and tailored solutions that align with industry best practices, enhancing the overall security posture of organizations utilizing generative AI technologies.
In conclusion, evaluating AI guardrails with sophisticated testing frameworks is a crucial step for any organization aiming to secure its generative AI systems. By leveraging tools like Microsoft’s PYRIT alongside other frameworks, companies can significantly reduce their vulnerability to emerging threats.
Future Directions: Ensuring Robustness in Generative AI Security
The landscape of generative AI systems is continuously evolving, demanding a proactive approach to security to safeguard against emerging threats. In this context, the persistent refinement of security tools is essential. Organizations must adopt a mindset of continuous improvement, revisiting and updating their security protocols as new vulnerabilities emerge. As generative AI technologies become more sophisticated, the potential attack vectors will also multiply, necessitating an adaptive security posture that evolves alongside these advancements.
Furthermore, collaboration is fundamental in the realm of generative AI security. By actively engaging with open source communities, companies can leverage collective expertise and insights to enhance their security frameworks. Such collaboration fosters an environment where businesses can share knowledge about threats, best practices, and innovative tools, ensuring that the security measures employed are not only up-to-date but also robust against future challenges. Open source tools allow for transparency and peer validation, serving as a critical line of defense against potential exploits.
Data plays a dual role in the context of generative AI; it is both a valuable asset and a possible attack vector. This duality underscores the importance of incorporating stringent security measures throughout every phase of AI development. From data collection and preprocessing to model training and deployment, each stage must include security considerations to protect sensitive information and maintain integrity. Implementing access controls, encryption, and regular audits can help mitigate risks associated with data-related vulnerabilities.
In summary, ensuring the robustness of generative AI security systems requires ongoing innovation, vigilance against emerging threats, and a commitment to collaboration within open source communities. By integrating security measures at the core of AI development, organizations can create resilient frameworks that not only defend against current risks but also adapt to future challenges in the ever-evolving realm of generative AI.
Discover more at InnoVirtuoso.com
I would love some feedback on my writing so if you have any, please don’t hesitate to leave a comment around here or in any platforms that is convenient for you.
For more on tech and other topics, explore InnoVirtuoso.com anytime. Subscribe to my newsletter and join our growing community—we’ll create something magical together. I promise, it’ll never be boring! 🙂
Stay updated with the latest news—subscribe to our newsletter today!
Thank you all—wishing you an amazing day ahead!